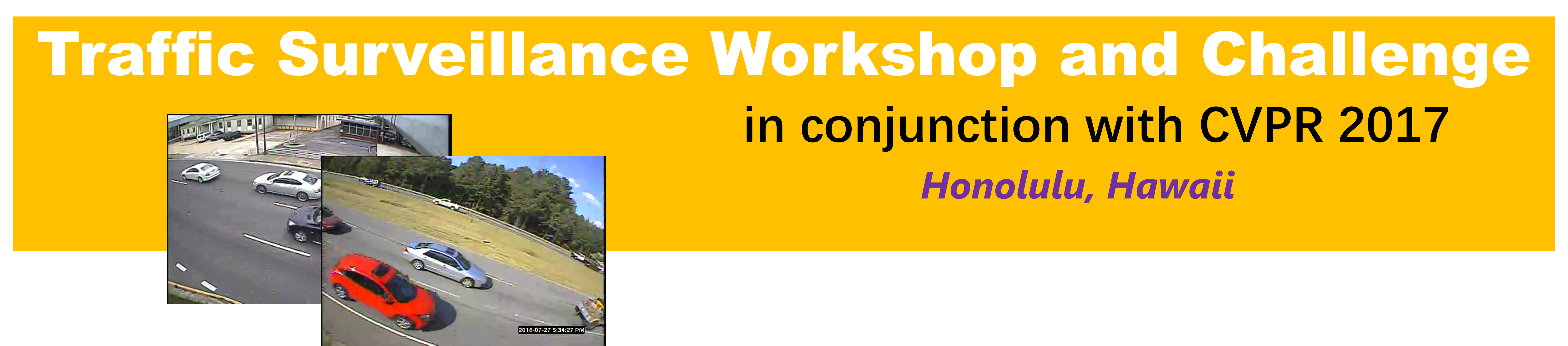
Workshop Overview:
The socio-economic costs of traffic accidents constitute a significant burden on the society and therefore decision-makers focus on reducing these numbers. In the EU, for example, the objective is to reduce the number of fatalities by 50% by 2020. In order to realize this ambitious goal a range of research directions must be undertaken, from general traffic understanding and analysis to building real-time surveillance systems. The proposed workshop is a step in this direction. The focus of this workshop is the analysis, implementation and deployment of automatic traffic surveillance systems in support of detecting, tracking and, in general, understanding the behavior of road-users.
Original research papers related to the following (or similar) topics are welcome:
- Detection, localization and classification of vehicles.
- Behavior understanding of road-users.
- Multi-sensor approaches to the above topics (for example: visual light combined with Lidar)
- User-centric approaches to the above topics (for example: vehicle-mounted sensing)
- Employment of systems into real-life setups (theoretical and practical challenges)
- Automatic understanding of the environment in traffic scenarios (for example: road signs, traffic light, lanes)
- Automatic individual and group behaviour interpretation (for example: unsafe behaviours, safety indicators and conflict detection)
- Applications related to traffic surveillance
* Note that a $1000 award sponsored by Atki will be delivered to the best paper.
The MIOvision Traffic Camera Dataset (MIO-TCD) Challenge:
In order to provide a framework for rigorous comparison of algorithms, we also propose a challenge on vehicle localization and classification. The challenge will be organized around a new traffic dataset, prepared and hosted at the University of Sherbrooke and Miovision Inc. Canada. The dataset consists of 786676 images acquired at different times of the day during different seasons by thousands of traffic cameras in Canada and the United States. The images have been selected to cover a wide range of challenges and are representative of typical visual data captured today in urban and rural traffic scenarios. Each foreground object (vehicles of all kinds as well as pedestrians and bicycles) has been carefully identified to enable a quantitative comparison and rigorous ranking of algorithms.
As mentioned in the DATASET page, the dataset comes with two parts : the “classification challenge dataset” and the “localization challenge dataset”. The best-performing methods submitted to the challenge will be invited for presentation at the workshop as regular talks, while all methods submitted will be reported on our on-line system as well as in a follow-up journal survey paper.
- The MIO-TCD dataset as well as some useful code can be download on the DATASET page
Important dates:
|
Paper submission:
- Prospective authors of a regular workshop paper are invited to submit a paper describing their contribution and results, including figures, tables, and references, by the due date (please see Key Dates above) at the TSWC-2017 submission site (Microsoft CMT)
- Prospective authors of a challenge paper are invited to submit a 4 to 8 pages long paper describing methodology and results, including figures, tables, and references, by the due date (please see Key Dates above) at the TSWC-2017 submission site (Microsoft CMT)
- Each submission must be formatted for double-blind review using one of the templates available at the CVPR 2017 Author Guidelines page
- Submissions not using the above templates or disclosing identity of the authors will be rejected without review.
- A paper submission implies that, if the paper is accepted, one of the authors, or a proxy, will present the paper at the workshop.
- The workshop proceedings will be published together with the main conference proceedings.
Invited Speakers:
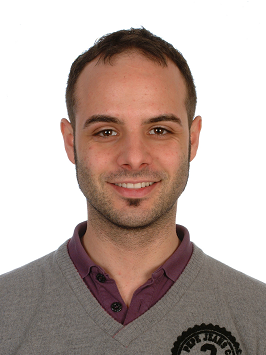
Prof. David Vazquez
Universitat Autònoma de Barcelona, Spain
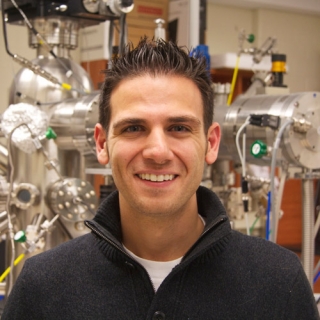
Dr. Andrew Achkar
Miovision Technologies Inc., Waterloo, Canada
Program
|
Organizers:
- Pierre-Marc Jodoin (Université de Sherbrooke, Canada)
Home page: http://info.usherbrooke.ca/pmjodoin - Justin Eichel (Miovision Technologies Inc., Canada)
- Andrew Achkar (Miovision Technologies Inc., Canada)
- Thomas B. Moeslund (Aalborg University, Denmark)
- Janusz Konrad (Boston University, USA)
Home page: http://sites.bu.edu/jkonrad - Akshaya Mishra (Miovision Technologies Inc., Canada)
- Shaozi Li (Xiamen University, China)
- Kalle Åström (Lund University, Sweden)
- Zhiming Luo (Université de Sherbrooke, Canada)
Acknowledgment:
- Yi Wang, Ph.D student, Université de Sherbrooke, Canada
Webmaster, software developer
- Yubin Lin and Chengji Wang (Xiamen University, China)
Help with ground truthing
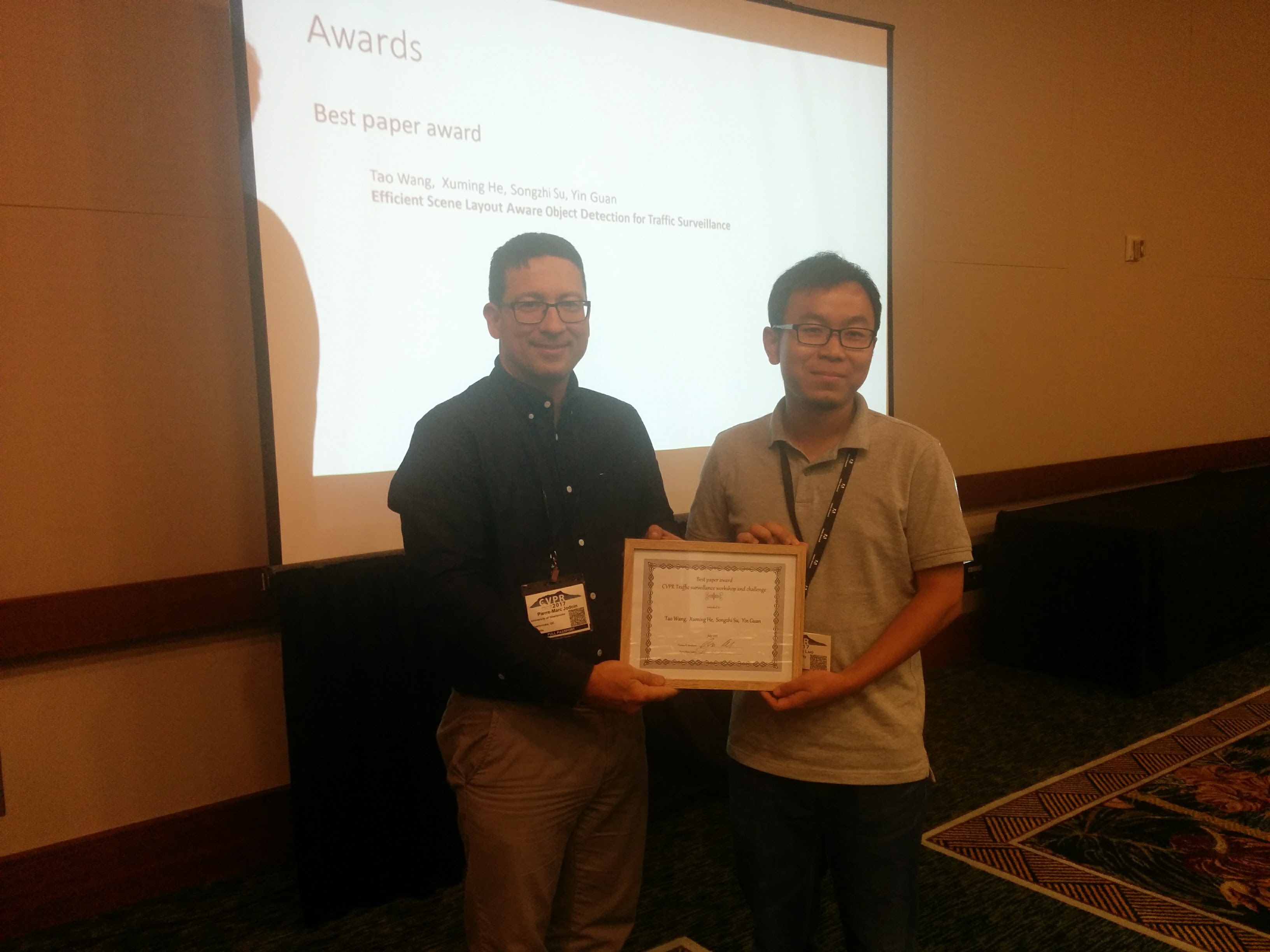
The winners of both 2017 mio-tcd challenges were Heechul Jung, Min-Kook Choi, Jihun Jung, Jin-Hee Lee, Soon Kwon, Woo Young Jung with their paper entitled "ResNet-based Vehicle Classification and Localization in Traffic Surveillance Systems"
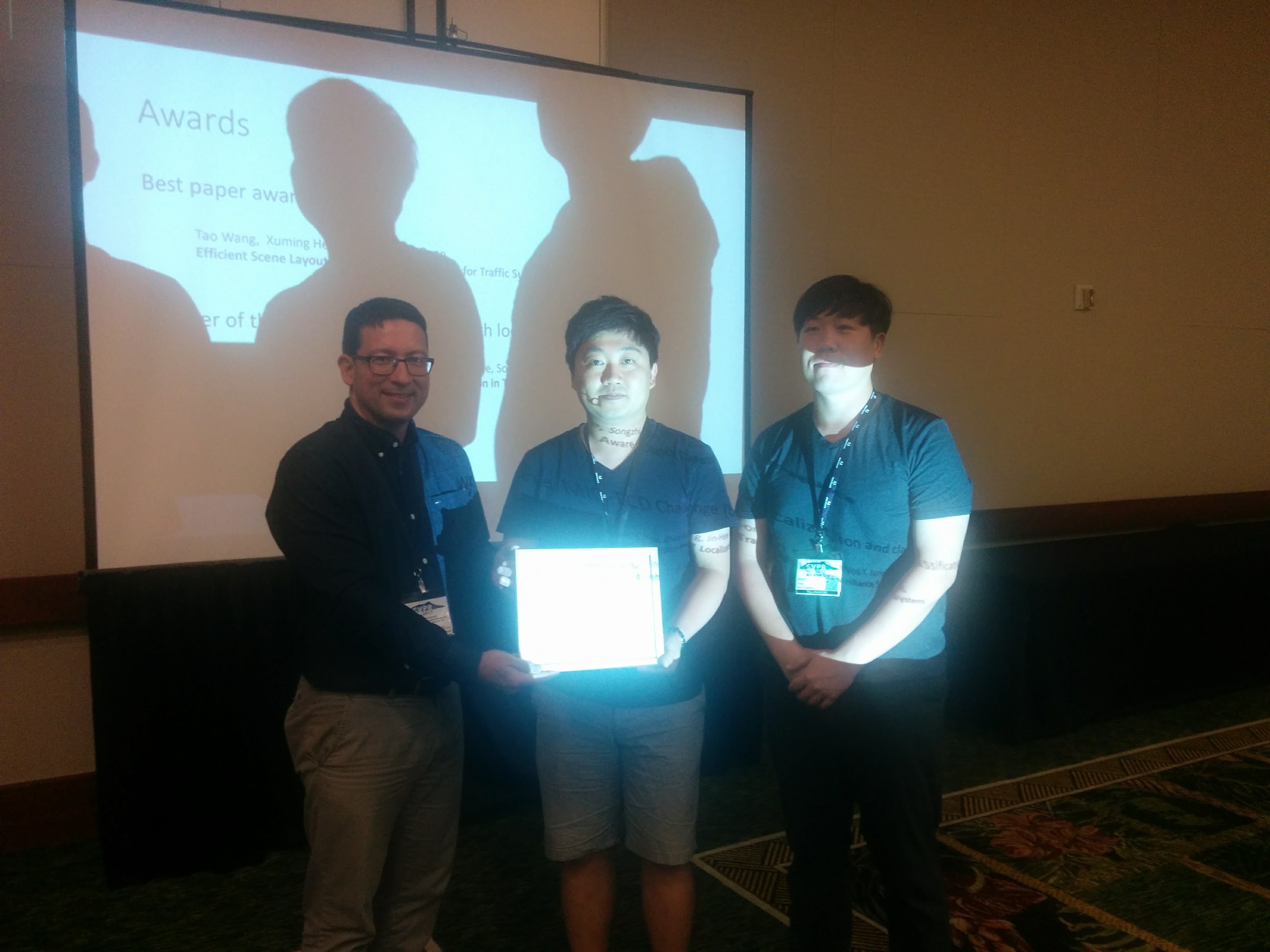
Challenge results on MIO-TCD dateset:
Results on the classification challenge
Method | Cohen Kappa Score | Accuracy | Mean Precision | Mean Recall | Articulated Truck | Bicycle | Bus | Car | Motorcycle | Non-motorized Vehicle | Pedestrian | Pickup Truck | Single Unit Truck | Work Van | Background |
---|---|---|---|---|---|---|---|---|---|---|---|---|---|---|---|
(C) Joint fine-tuning with DropCNN [165] | 0.9681 | 0.9795 | 0.9530 | 0.8970 | 0.9324 | 0.8949 | 0.9779 | 0.9853 | 0.9111 | 0.5228 | 0.9406 | 0.9539 | 0.8336 | 0.9166 | 0.9984 |
(C) Ensemble of Local Expert and Global Networks [166] | 0.9675 | 0.9792 | 0.9298 | 0.9024 | 0.9358 | 0.8774 | 0.9620 | 0.9889 | 0.9212 | 0.6872 | 0.9425 | 0.9507 | 0.8289 | 0.8353 | 0.9966 |
(C) bagging + CNN [167] | 0.9666 | 0.9786 | 0.9355 | 0.9041 | 0.9412 | 0.8739 | 0.9593 | 0.9866 | 0.9131 | 0.7078 | 0.9610 | 0.9510 | 0.8273 | 0.8258 | 0.9980 |
(C) Ensemble of Deep Networks: Network A,B and C [168] | 0.9658 | 0.9780 | 0.9439 | 0.9190 | 0.9451 | 0.8984 | 0.9794 | 0.9790 | 0.9374 | 0.7237 | 0.9348 | 0.9624 | 0.8445 | 0.9059 | 0.9980 |
Results on the localization challenge
Method | Mean Average Precision | Articulated Truck | Bicycle | Bus | Car | Motorcycle | Motorized Vehicle | Non-motorized Vehicle | Pedestrian | Pickup Truck | Single Unit Truck | Work Van |
---|---|---|---|---|---|---|---|---|---|---|---|---|
SSD-300 [5] | 0.7397 | 0.9063 | 0.7834 | 0.9568 | 0.9145 | 0.7891 | 0.5144 | 0.5522 | 0.3730 | 0.9068 | 0.6904 | 0.7496 |
YOLO-V1 [8] | 0.6265 | 0.8272 | 0.7002 | 0.9156 | 0.7716 | 0.7143 | 0.4441 | 0.2068 | 0.1808 | 0.8559 | 0.5830 | 0.6926 |
Faster-RCNN [9] | 0.6998 | 0.8593 | 0.7836 | 0.9518 | 0.8259 | 0.8106 | 0.5280 | 0.3743 | 0.3125 | 0.8903 | 0.6249 | 0.7364 |
YOLO-V2-PascalVOC [53] | 0.7147 | 0.8674 | 0.7839 | 0.9521 | 0.8051 | 0.8086 | 0.5195 | 0.5645 | 0.2573 | 0.8459 | 0.7000 | 0.7570 |
YOLO-V2-MIOTCD [55] | 0.7183 | 0.8831 | 0.7864 | 0.9513 | 0.8136 | 0.8136 | 0.5170 | 0.5657 | 0.2496 | 0.8648 | 0.6923 | 0.7643 |
SSD-512 [57] | 0.7732 | 0.9213 | 0.7859 | 0.9679 | 0.9402 | 0.8233 | 0.5675 | 0.5882 | 0.4356 | 0.9307 | 0.7401 | 0.8039 |
(L) RFCN-ResNet_ensemble4 [58] | 0.7924 | 0.9248 | 0.8734 | 0.9746 | 0.8970 | 0.8821 | 0.6232 | 0.5909 | 0.4857 | 0.9225 | 0.7442 | 0.7986 |
(L) ContextModelA [59] | 0.7719 | 0.9162 | 0.7990 | 0.9677 | 0.9380 | 0.8363 | 0.5640 | 0.5823 | 0.4261 | 0.9275 | 0.7380 | 0.7956 |